Data Scientist Salary
Updated on
The key determinant for a lucrative data science salary is primarily its geographic source, with the United States often offering the most competitive rates. In the past, this typically necessitated relocation. However, the current landscape offers increasingly more remote opportunities, revolutionizing the way you approach your career trajectory. The crucial question to consider now is: "How can I work for a US based company?".
If you'd like to check how much you should earn as a Data Scientist / ML Engineer, you might want to check our Salary calculator for Data Scientists.
Overall statisics
The median annual compensation (salary + bonus + equity) of a Data Scientist is 22,500 USD worldwide, according to the data from Kaggle 2022 Machine Learning and Data Science survey. The top 10% Data Scientists make above 137,500 USD annually, while the Top 1% make above 400,000 USD annually.
The findings in this article are based on a Data Science compensation prediction model which was built by a Kaggle Competitions Grandmaster narsil for the 2022 Kaggle Machine Learning & Data Science Survey - Analytics competition. It received an Honourable Mention from competition hosts and was in the Top 9 among more than 300 submitted solutions.
Key remarks about the Data Science salary prediction model
- The model is based on data from 294 questions and 23,997 responses in a 2022 Kaggle Machine Learning & Data Science Survey, combined with external data.
- The model identifies 6 key features impacting the total compensation of Data Science Professionals, which together preserve 75% of the signal of the original 294 questions. Those 6 key features are included as the drop-downs in the calculator above.
- The model is bad at predicting very high salaries. It will not show you how to make a million dollars per year with Data Science. However, it shows reasonable salary benchmarks for entry, mid, senior and manager Data Scientists.
You can find the full documentation of the model, together with community discussion here.
Which factors affect the salary of a Data Scientist?
The most important factors driving the Data Scientist's salary, listed by their importance:
- Country
- Industry
- Job title (specific skill set)
- Years of Coding experience
- Years of Machine Learning experience
We measured the importance of all of the factors in two steps:
- Feature importance: gain taken directly from the LGBM model
- Percent error reduction vs simple baseline when such a feature is removed from the model. This is our custom metric, built specifically to measure the importance of all the features in explaining the compensation of Data Scientists.
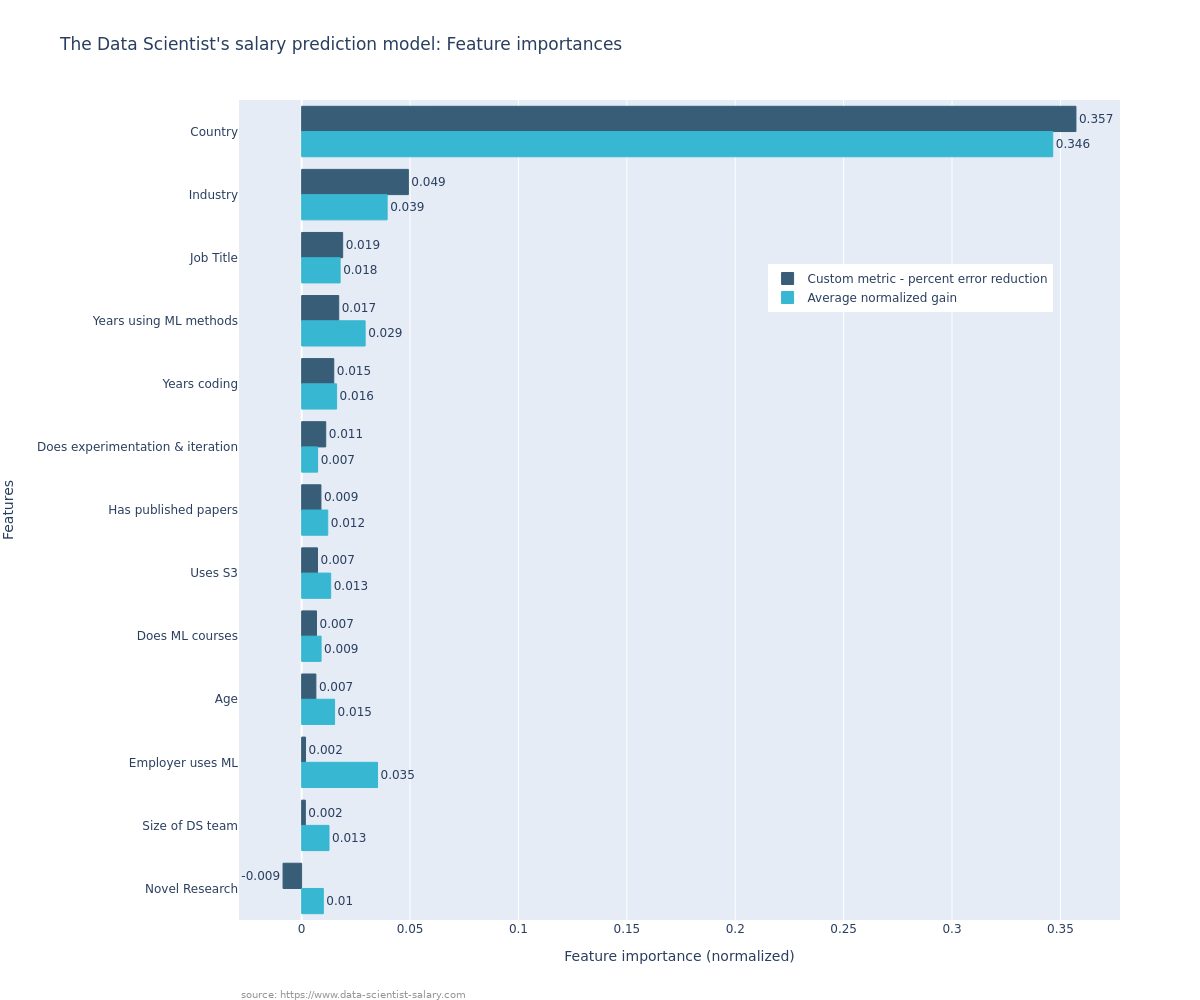
Key conclusions:
- The most important factor driving the Data Scientist's salary is the country of work. It is ~10x more important than other factors. This has serious implications for the optimal strategy to increase your Data Scientist salary, as we explore below.
- The second most important factor for a Data Science salary is the Industry. The main reason is that different industries generate different value from Data Science work. Industries with more data (especially labeled data) get higher Returns on Investment from Data Science work and offer higher salaries to Data Scientists.
- The third most important factor for a Data Science salary is the Job Title, which describes the scope of responsibilities and skills required to perform the role.
- The fourth and fifth most important factors for a Data Science salary are years of experience in coding and applying ML methods.
Let's explore the exact relationship between all of those factors and a Data Scientist's salary.
Data Scientist Salary by Country
Country of work is the most important factor impacting the Data Scientist's Salary. The highest-paying countries in Data Science are:- United States of America, with the average Data Science compensation of 134k USD
- Australia, with an average Data Science compensation of 121k USD
- Israel, with an average Data Science compensation of 119k USD
These 3 countries on average offer significantly higher Data Science salaries than other countries.
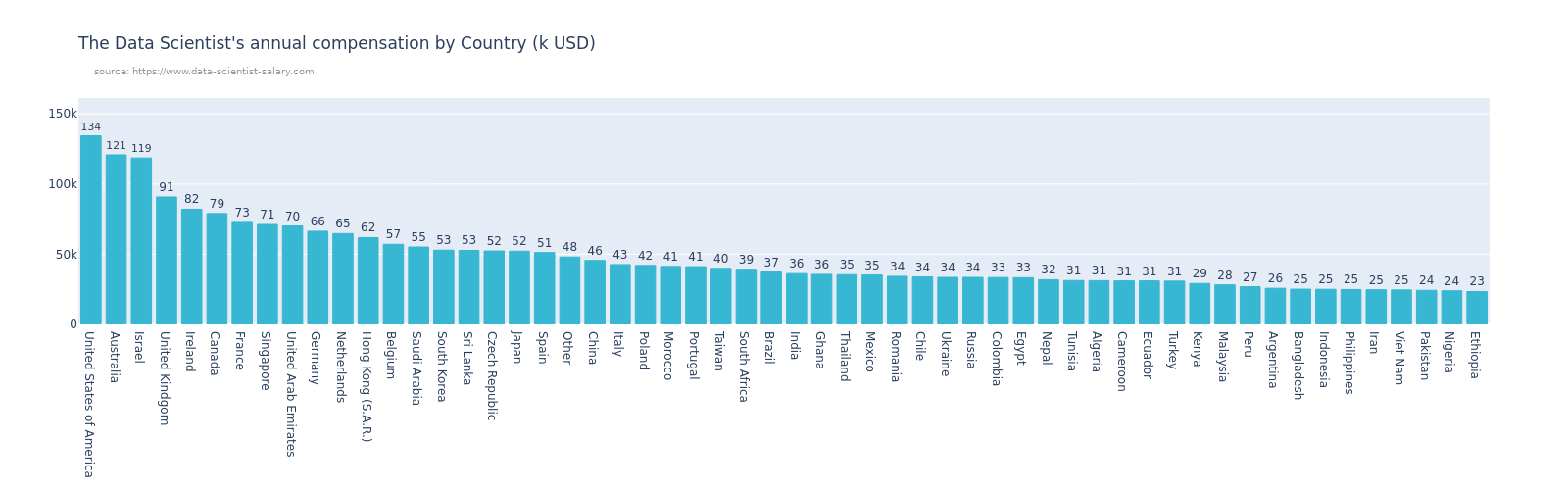
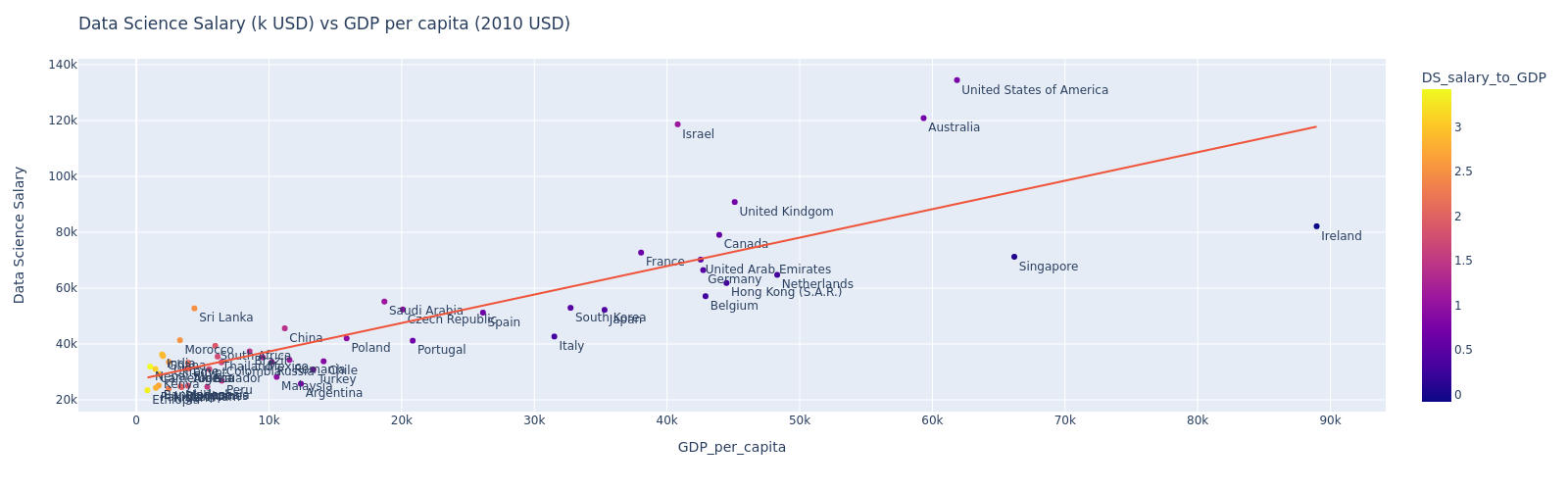
Data Scientist Salary by City in United States
Analytics in this section was prepared by Miłosz Goszczyński.
The geographical variance is not just apparent between countries but also within the borders of a single country, such as the United States, where the differences can be strikingly large. An analysis of the top cities for data science salaries within the U.S. reveals an interesting pattern, primarily concentrated around high-cost living areas but also showing pockets of high earning potential outside these regions.
Leading the pack is Santa Clara, with an impressive annual data science salary of $207,125 and a robust job market featuring 537 job offers. Its position is bolstered by the area's vibrant tech ecosystem and high salary offerings, which, despite high taxes and living costs, allow for substantial annual savings of $103,150. Not far behind, South San Francisco and Palo Alto offer competitive salaries and savings opportunities, though with a smaller number of job openings.
Interestingly, cities like Hillsboro and Bentonville, traditionally not seen as tech hubs, have made significant leaps in the rankings. Hillsboro, in particular, comes in fifth with an annual data science salary of $141,000 and annual savings of $75,194, thanks to the presence of Intel. The tech giant has played a pivotal role in transforming Hillsboro into a burgeoning center for tech talent, boosting its desirability for data scientists looking for promising career opportunities in less conventional locales. Similarly, Bentonville, home to Walmart's headquarters, ranks ninth with an annual salary of $135,000 and savings of $70,440. Walmart's investment in data analytics and technology innovation has propelled Bentonville up the rankings, underscoring the growing importance of retail giants in the tech landscape.
The full ranking is presented below.
Notes:
- In this analysis, we focused on salary data extracted from job listings on the Job Hunter website. In many U.S. states, pay transparency laws mandate that companies reveal the salary range for positions. To conduct our study, we calculated the midpoint of these salary ranges by adding the lower and upper limits and dividing by two. We then determined the median salary for each city by analyzing these midpoint figures.
- Annual salary is the median midpoint (average of low and high range) salary quoted in job offers indexed by the Job Hunter.
- Taxes calculated by Chat GPT
- Cost of living from https://livingwage.mit.edu/ (for 1 Adult 0 Children), and https://livingcost.org/ as a fallback source. Where MIT data was unavailable we took the data from https://livingcost.org/ and adjusted for median under-prediction vs MIT.
- N job offers is the number of job offers with quoted salary. On average 10-20% of offers contain the salary range.
Rank | City | Annual Salary | Taxes | Annual Cost of Living | Annual Savings | N job offers |
---|---|---|---|---|---|---|
1 | Santa Clara | 207125 | 46566 | 57409 | 103150 | 537 |
2 | South San Francisco | 198625 | 43983 | 52907 | 101735 | 95 |
3 | Palo Alto | 182250 | 39216 | 58746 | 84288 | 74 |
4 | Sunnyvale | 175500 | 37517 | 57219 | 80764 | 185 |
5 | Hillsboro | 141000 | 28824 | 36983 | 75194 | 54 |
6 | San Bruno | 160000 | 33563 | 52823 | 73614 | 92 |
7 | San Jose | 165350 | 34811 | 57219 | 73320 | 376 |
8 | San Francisco | 153550 | 32281 | 50504 | 70765 | 1034 |
9 | Bentonville | 135000 | 27946 | 36614 | 70440 | 41 |
10 | Redwood City | 160000 | 33563 | 56363 | 70074 | 51 |
11 | Milwaukee | 130815 | 27110 | 34090 | 69615 | 47 |
12 | Austin | 128800 | 26674 | 35014 | 67112 | 369 |
13 | Cambridge | 150110 | 31521 | 52155 | 66434 | 48 |
14 | Alameda | 147500 | 31099 | 50199 | 66202 | 48 |
15 | Birmingham | 130000 | 26991 | 37246 | 65763 | 78 |
16 | Rahway | 138500 | 28712 | 45423 | 64365 | 116 |
17 | Princeton | 135000 | 27946 | 44022 | 63032 | 67 |
18 | Seattle | 142500 | 29900 | 51452 | 61148 | 446 |
19 | Pleasanton | 154250 | 32489 | 60693 | 61068 | 72 |
20 | Columbia | 123188 | 25417 | 37255 | 60515 | 97 |
21 | Livermore | 140280 | 29174 | 50642 | 60465 | 228 |
22 | Charlotte | 125930 | 26052 | 40756 | 59122 | 182 |
23 | Irving | 116500 | 24164 | 33241 | 59096 | 293 |
24 | Annapolis Junction | 133900 | 27858 | 47722 | 58320 | 165 |
25 | Bellevue | 137675 | 28578 | 51452 | 57646 | 98 |
26 | Herndon | 125350 | 25936 | 42201 | 57213 | 88 |
27 | Plano | 125930 | 26052 | 42688 | 57190 | 75 |
28 | Houston | 117500 | 24370 | 36446 | 56684 | 135 |
29 | Dallas | 121445 | 25062 | 39901 | 56482 | 351 |
30 | Atlanta | 125968 | 26099 | 44862 | 55007 | 384 |
31 | Ann Arbor | 120000 | 24720 | 40904 | 54376 | 64 |
32 | Bethesda | 125000 | 25864 | 45054 | 54082 | 251 |
33 | Folsom | 126000 | 26114 | 45901 | 53986 | 69 |
34 | O'Fallon | 112000 | 23336 | 36545 | 52119 | 103 |
35 | Durham | 117500 | 24370 | 41651 | 51480 | 43 |
36 | Huntsville | 112000 | 23336 | 37284 | 51380 | 134 |
37 | Greenwood Village | 103850 | 21992 | 33929 | 47929 | 68 |
38 | Jersey City | 121000 | 25016 | 48868 | 47116 | 141 |
39 | Phoenix | 114500 | 23840 | 43833 | 46828 | 121 |
40 | Hampton | 107250 | 22551 | 37884 | 46815 | 45 |
41 | Los Angeles | 117500 | 24370 | 47067 | 46064 | 411 |
42 | Baltimore | 103000 | 21358 | 35934 | 45708 | 89 |
43 | Rockville | 107450 | 22565 | 39198 | 45688 | 52 |
44 | Manhattan Beach | 102240 | 21222 | 35569 | 45449 | 75 |
45 | Arlington | 118522 | 24521 | 48923 | 45078 | 476 |
46 | Miami | 105000 | 21826 | 38478 | 44696 | 52 |
47 | New York | 115000 | 23766 | 46584 | 44650 | 2457 |
48 | Minneapolis | 107000 | 22556 | 40376 | 44068 | 199 |
49 | Cincinnati | 100000 | 20795 | 35296 | 43909 | 48 |
50 | Boulder | 113725 | 23621 | 46197 | 43907 | 42 |
51 | Portland | 108300 | 22696 | 41707 | 43896 | 155 |
52 | Portage | 98850 | 20496 | 35016 | 43338 | 43 |
53 | Harrison | 113000 | 23498 | 46245 | 43256 | 51 |
54 | Aurora | 100000 | 20795 | 36586 | 42619 | 83 |
55 | Tampa | 105515 | 21892 | 41022 | 42601 | 476 |
56 | Colorado Springs | 104475 | 21799 | 40626 | 42050 | 243 |
57 | Springfield | 95700 | 19734 | 34923 | 41043 | 130 |
58 | Santa Ana | 105000 | 21826 | 42252 | 40922 | 49 |
59 | Hartford | 101250 | 21039 | 39989 | 40222 | 153 |
60 | Clearfield | 93200 | 19346 | 33776 | 40078 | 53 |
61 | Boston | 116250 | 24075 | 52155 | 40020 | 375 |
62 | Frederick | 107250 | 22551 | 44819 | 39880 | 43 |
63 | Salt Lake City | 100550 | 20890 | 39934 | 39726 | 78 |
64 | Ashburn | 111000 | 23086 | 48208 | 39705 | 54 |
65 | Chicago | 102500 | 21264 | 41980 | 39256 | 435 |
66 | Anaheim | 110834 | 23034 | 48615 | 39185 | 60 |
67 | Indianapolis | 97500 | 20246 | 38070 | 39184 | 101 |
68 | Deerfield | 96000 | 19971 | 37000 | 39029 | 75 |
69 | Melbourne | 95650 | 19831 | 36819 | 39000 | 131 |
70 | Eden Prairie | 100450 | 20879 | 40641 | 38930 | 62 |
71 | Fort Meade | 126800 | 26223 | 62471 | 38106 | 165 |
72 | Alexandria | 107150 | 22444 | 46612 | 38094 | 105 |
73 | Roy | 93200 | 19346 | 36261 | 37592 | 110 |
74 | Washington | 105500 | 21951 | 46059 | 37490 | 731 |
75 | Golden | 94450 | 19612 | 37508 | 37330 | 63 |
76 | Campbell | 93150 | 19325 | 36720 | 37105 | 98 |
77 | San Antonio | 93605 | 19448 | 37117 | 37040 | 142 |
78 | Santa Monica | 121575 | 25114 | 59854 | 36607 | 71 |
79 | Jacksonville | 95640 | 19830 | 39879 | 35931 | 105 |
80 | Newport Beach | 127900 | 26436 | 65559 | 35905 | 67 |
81 | Columbus | 85300 | 17596 | 31801 | 35904 | 198 |
82 | Plymouth | 107000 | 22556 | 48751 | 35692 | 40 |
83 | Charlottesville | 95650 | 19831 | 40286 | 35533 | 75 |
84 | Waltham | 104712 | 21775 | 47537 | 35401 | 40 |
85 | Suffolk | 95650 | 19831 | 40795 | 35024 | 41 |
86 | Palmdale | 99950 | 20733 | 44467 | 34750 | 76 |
87 | Reston | 101462 | 21097 | 45624 | 34740 | 273 |
88 | New Castle | 94325 | 19551 | 40915 | 33859 | 46 |
89 | San Diego | 107900 | 22464 | 51796 | 33640 | 503 |
90 | Englewood | 91153 | 18955 | 39349 | 32848 | 65 |
91 | Madison | 86750 | 17914 | 36609 | 32227 | 43 |
92 | Milpitas | 105000 | 21826 | 51598 | 31576 | 72 |
93 | Scottsdale | 90500 | 18776 | 41245 | 30480 | 82 |
94 | Denver | 95000 | 19716 | 44968 | 30316 | 433 |
95 | Newark | 98148 | 20390 | 48868 | 28890 | 121 |
96 | Oklahoma City | 77725 | 15877 | 34250 | 27598 | 48 |
97 | Philadelphia | 83932 | 17362 | 39294 | 27277 | 86 |
98 | St. Louis | 77562 | 15843 | 34736 | 26984 | 208 |
99 | Las Vegas | 85000 | 17520 | 41023 | 26457 | 57 |
100 | Odenton | 109500 | 22666 | 60407 | 26426 | 77 |
101 | Chantilly | 133900 | 27858 | 79620 | 26421 | 150 |
102 | Richmond | 100418 | 20850 | 53213 | 26355 | 79 |
103 | Omaha | 80000 | 16520 | 37271 | 26208 | 43 |
104 | Norfolk | 104825 | 21779 | 57442 | 25604 | 76 |
105 | Irvine | 96900 | 20056 | 52991 | 23853 | 175 |
106 | Louisville | 72500 | 15102 | 33862 | 23537 | 57 |
107 | Chandler | 84700 | 17449 | 43833 | 23418 | 41 |
108 | Woonsocket | 74400 | 15388 | 35791 | 23221 | 49 |
109 | Saint Paul | 73260 | 15152 | 35204 | 22903 | 45 |
110 | Dulles | 107900 | 22464 | 63663 | 21773 | 47 |
111 | Camden | 79800 | 16428 | 41917 | 21454 | 43 |
112 | Redondo Beach | 106200 | 22076 | 63126 | 20998 | 121 |
113 | El Segundo | 101654 | 21043 | 59870 | 20741 | 121 |
114 | Burlington | 83279 | 17239 | 45320 | 20720 | 55 |
115 | Hoover | 72551 | 15107 | 37246 | 20198 | 41 |
116 | Linthicum Heights | 104000 | 21606 | 62471 | 19922 | 94 |
117 | McLean | 124000 | 25696 | 79771 | 18532 | 792 |
118 | Culver City | 82550 | 17020 | 47705 | 17825 | 45 |
119 | Burbank | 89710 | 18557 | 54333 | 16821 | 63 |
120 | Fort Mill | 100050 | 20792 | 62555 | 16702 | 64 |
121 | Quantico | 112000 | 23336 | 74721 | 13943 | 41 |
122 | Fort Belvoir | 99000 | 20490 | 79771 | -1261 | 67 |
123 | Getzville | 64215 | 13216 | 53024 | -2026 | 135 |
Data Scientist Salary by Industry
The industry is the second most important factor driving the salary of a Data Scientist. The top paying industries in Data Science are:
- Accounting/Finance with the average Data Science compensation of 57k USD annually.
- Online Services/Internet Based Services with an average Data Science compensation of 52k USD annually.
- Computers/Technology with the average Data Science compensation of 50k USD annually.
- Broadcasting/Communication with the average Data Science compensation of 49k USD annually.
- Marketing/CRM with the average Data Science compensation of 48k USD annually.
- Medical/Pharmaceutical with the average Data Science compensation of 47k USD annually.
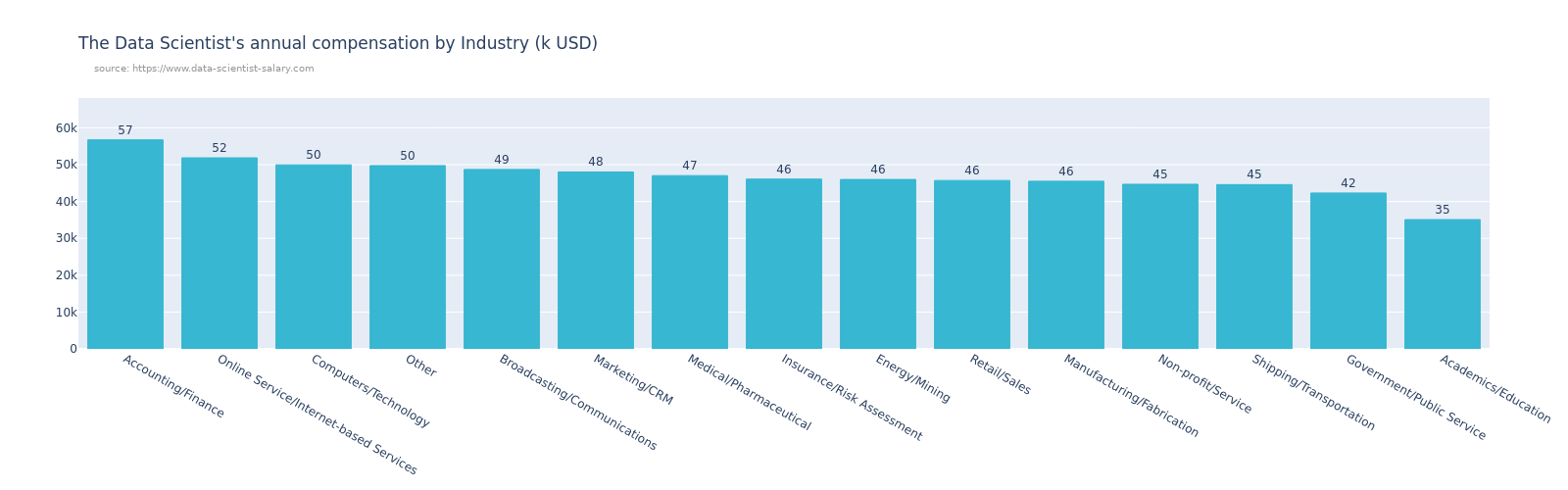
When we see that the Data Science salaries are the highest for the banks, online services sector, and technology companies, we tend to resort to some sort of a mental shortcut:
"Yes, these are Morgan Stanleys of this world and FAANG companies, they have tons of money and can shower everyone with dollars".
What we often forget is that these are extremely smart organizations, listed on stock exchanges, with very strong CFO mandates, and answering directly to their shareholders.
TL;DR: they would not pay as high if they did not see the value attached.
The point is that the Data Science salaries in Banks/Tech/Online sectors are high, because the value of Data Science work is high in those sectors (higher than in other sectors). It is the same effect that we observe at the country level - where the salary is closely tied to GDP. On an industry level, the Data Science salary is tied to its role and importance in creating value. The key drivers are the following:
- The Data Culture. Banks, the online industry & tech companies were born based on data - it pervades (almost) every aspect of their operations.
- Large volumes of labeled, structured, and clean data available for modeling.
- Ability for quick A/B testing of any idea, and hence a very quick iteration cycle, much quicker compared to other industries.
- Network effects and self-reinforcement: because of the factors above, such sectors attract top Data Science talents who reinforce the above points, and over time they drive even more value.
Data Professionals Salary Progression by Job Title
Analytics in this section was prepared by Miłosz Goszczyński.
Job Title, which is also a description of the skillset required to perform a role is the third most important factor driving the salary of a Data Scientist. Overall, the most profitable professions are:
- Machine Learning / ML Ops roles with Median Salary for Senior Roles around $160k.
- Data Architect roles with Median Salary for Senior Roles around $150k.
- Software Engineer in Machine Learning roles with Median Salary for Senior Roles around $135k.
Because of the importance of choosing the right career track, we have analyzed not only the overall salary levels per profession, but also the salary progression:
Data Administrators see a significant salary increase as they move up from junior to manager roles, with a notable $31.5k jump from junior to regular levels and a $32.5k increase from senior to manager roles, without an increase towards Director / VP roles. This suggests strong demand and competitive pay up to the managerial level, followed by a plateau as roles shift from technical to more strategic responsibilities.
Data Analysts experience steady salary growth throughout their career progression, starting with a $19.2k increase from junior to regular levels, followed by a $15.4k rise to senior positions. The most substantial jump occurs at the managerial level, with a $32.5k increase, reflecting the heightened responsibilities and expertise required. The transition from Manager/Lead to Director/VP sees a modest $2.7k increase, indicating that the highest value is placed on the managerial expertise rather than strategic oversight.
Data Architects start with a higher median salary and see substantial growth, particularly from junior to regular roles, with a significant $67.5k increase. The salary growth continues steadily, with a $17.6k rise to senior levels and a slight $0.3k increase to managerial roles, suggesting a plateau in salary growth at this stage. However, the peak occurs at the Director/VP level with a $25.2k increase, highlighting the value placed on high-level strategic and architectural oversight.
Data Engineers enjoy a progressive salary increase across all seniority levels, starting with a $36.7k increase from junior to regular roles, followed by a $22.5k rise to senior positions. The most significant growth happens at the managerial level, with a $38.2k jump, reflecting the critical nature of leadership in engineering projects. The highest median salary is at the Director/VP level, with an additional $19.2k increase, underscoring the strategic importance of data engineering leadership.
Data Scientists' salaries increase steadily with seniority, with a $32.4k jump from junior to regular levels and a $25.6k increase to senior positions. The transition to managerial roles sees a $20.5k rise, with a further $24.4k increase at the Director/VP level. The steady growth highlights the consistent demand for advanced analytical skills and the value placed on leadership in data science.
Machine Learning/MLOps Engineers command high salaries across all levels, with a remarkable $69.0k increase from junior to regular positions, and a $20.4k rise to senior roles. Managerial positions see a $19.8k increase, while the Director/VP level commands the highest median salary in this dataset with a $28.9k rise. This trend emphasizes the high demand and value of expertise in deploying and managing machine learning models at all levels, particularly at the executive level.
Research Scientists have a steady salary increase, starting with a $26.0k rise from junior to regular levels, and a $27.8k increase to senior positions. The progression to managerial roles sees a $26.2k rise, with a marginal $1.0k increase at the Director/VP level. This pattern indicates consistent value placed on research expertise and leadership, with a slight plateau at the executive level.
Software Engineers see progressive salary growth throughout their career, with a notable $40.5k increase from junior to regular roles and a $25.1k rise to senior positions. The managerial level experiences a significant $38.0k increase, reflecting the critical role of leadership in software development. However, there’s a slight decrease of $34.1k at the Director/VP level, suggesting a shift in the valuation of technical versus strategic skills at the highest levels.
Statisticians start with lower salaries compared to other professions but see significant increases, particularly from the senior to Director/VP levels. The salary rises by $32.9k from junior to regular levels and by $33.1k to senior positions. Managerial roles see an $18.4k increase, with the most substantial jump of $48.2k at the Director/VP level. This trend underscores the growing importance and value of statistical expertise and leadership at higher levels of responsibility.
How to Increase Your Data Scientist Salary
These are the top 3 actions you can take to increase your Data Scientist's salary, based on our analyses above:
- Optimize the country! This is 10x more important than everything else. It does not really matter if you are a Top Data Scientist if you are employed in a low-paying country. Changing the nationality of your Data Science payroll does not necessitate a physical relocation since COVID changed everything for remote work.
- Optimize the industry. Pick one where Data Science is at the heart of the technology and solves an important problem.
- Decide if you want to be a manager or a technical expert. Adjust your project & career choices accordingly to gain relevant experience.
Let's consider those strategies in more detail.
How to optimize the country to increase a Data Scientist's salary
There are 3 main ways to change the country of origin of your Data Science Salary:1. Relocate to a given country and get a local Data Science position.
Since this requires a physical relocation, it is the most complicated approach because of the logistics involved. On the other hand, it brings about the benefit of being close to the product team and the executive team, which might be very beneficial to your career in the long term.2. Get a remote Data Science position in a company based in a high-paying country.
For this strategy you will need a constant inflow of remote Data Science job opportunities. The response rates to job applications without insider referrals are typically very low, so be prepared to send a lot of CVs to get even one interview. The correct strategy here involves a lot of patience:- Start by sending out 1-2 CVs per week to relevant positions, even when you are very satisfied with your current job. It does not hurt to have options, does it?
- Automate the search criteria to save time - you will be doing a lot of sending.
- Write a short cover message to attach to the application, and customize it for every application you send. This should increase response rates to your applications.
- Don't get discouraged even if the first 50 applications fall silent. Adjust your search criteria. Add diversity to the job offers you are considering and see what works.
As much as the process is lengthy (but not time-consuming if the search process is automated properly) - it is totally worth it when one looks at the salary figures in different countries.
An interesting strategy involves startups, which typically have a lot of money from their financing rounds, but very little time to deliver their KPIs to investors. They don't have that many policies in place and are often open to flexible/remote work. What they look for is talent that can solve their problem, hence, they are more willing to outsource the Data Science work to remote employees at good prices.
3. Get a Data Science position in the local office of an international company.
The largest Data Science organizations have already realized the disparity between salaries and talent levels in different countries. They set up local offices in low-paying countries to attract the best talent at good prices (compared to what they would have to pay in their origin countries). From a perspective of a local Data Scientist, this seems like a very attractive option since it entails working for a renowned international company without the need to relocate.
However, one needs to remember that these are very smart organizations, and they set up local offices to exactly counter what you want to achieve: have your Data Science salary based in a high-paying country. As a result of their salary optimization policy, the salary levels between offices are very different even within the same company. So even if you manage to land such a position, you might consider it as a starting point to physically relocate within the same company to a high-paying country or to keep on sending your CVs regularly for remote offers.
Remember, it does not hurt to have options, nor does it mean you are disloyal in any way to your current employer. We all just operate on a free market, and the best moment to search for a job is when you have a very good job already.
How to optimize the industry to increase a Data Scientist's salary
Just as there is a very high correlation between GDP per capita in a given country and the Data Scientist's salary, there is a correlation between the value generated by Data Science in a given industry and the salary of Data Scientists working there.
Data Scientists benefit a lot from industry knowledge, and consequently, industry choice is very important. ML practitioners need to spend a significant time learning the industry before they are able to truly shine with ML models. When thinking about which industry (or a specific company, as the same logic applies there) to choose for your Data Science career, consider the following questions:
-
How important is Data Science for a given industry (company)?
Industries and companies where Data Science solves an important problem are willing to pay more for Machine Learning and Data Science skills. An example of an industry where Data Science is at the heart of technology and solves a core problem is AdTech (programmatic ad buying). The fundamental factors of AdTech leading to a high value of the Data Science work are the following:
- Vast Scale. 100 billion requests/day with a latency below 10ms is not uncommon. The number, speed, and accuracy of decisions required can only be met with Machine Learning.
- Auctions. The industry is based on auctions, with the 'winner takes it all' mechanic. An improvement in the accuracy of the model by 1% leads to disproportionately large gains over the competition.
- Abundance of labeled data, allowing for complex feature engineering and modeling strategies.
Here is an article explaining in detail the value drivers of Data Science in Adtech.
When choosing the industry, a Data Scientist should consider if there are similar fundamental factors present, leading to a high value of the Data Science work.
-
How much benefit does a 5-10% improvement of an existing solution bring?
Machine Learning models typically improve and automate processes already existing in the business. An average improvement of 5 to 10% can normally be expected when deploying an ML model. Is it a lot, in a given industry/company? What does it mean for them? Industries and companies where a 5% improvement generates a lot of absolute dollar value tend to pay more for Data Science work.
-
Is the Data Science role more related to R&D, or does it solve an actual production-level problem?
If the role of Data Science is mostly R&D, it is the first role to optimize when times get rough and the last one to hire. Industries and companies where Data Science solves an important production-level problem are willing to pay more for Machine Learning and Data Science skills.
Top paying companies for Data Scientists
The presence of self-reporting salary platforms enables us to directly compare the top-paying companies in Data Science (but beware of the bias in self-reported salary data!). According to levels.fyi, these are the top-paying companies in Data Science:
- Netflix, with a median Data Science salary of 505k USD
- Roblox, with a median Data Science salary of 490k USD
- AirBnb, with a median Data Science salary of 440k USD
While such very high salaries might be available only in specific locations, they might serve as a useful signal when conducting your search for remote Data Science and Machine Learning offers.
Data Scientist Salary benchmarks by data source
Below we compare 4 reputable data sources for Data Science salaries: Glassdoor, Levels.fyi, Payscale and Kaggle. In general, they have the following characteristics:
- Levels.fyi seems to be the most inflated
- Glassdoor claims most often the largest sample-size
- Kaggle surveys can be a source of incremental information for countries like Poland, where the adoption of popular services like Glassdoor is low
However, when comparing different Data Science Salary sources, the most important observation for us is that differences in average Data Scientists' Salaries between different data sources are staggering, even within the same country.
We can see that for countries with lower sample sizes (e.g. China) the differences can be as much as 10x. However, even in countries where all sources have hundreds of reported salaries we observe up to 6x differences (like India).
For any Data Scientist, it is clear that such differences without explanation undermine the credibility of all data sources. Ultimately, which source is to be trusted? In our view, such differences are inevitable in self-reported data because the distribution of answers is impacted by the ability of those platforms to reach a specific target group. We describe below how to address this issue properly.
Avoiding common mistakes in Data Scientist Salary calculation methodology
A common mistake observed with various analyses related to Data Science salary, is taking the self-reported data at its face value and drawing wrong conclusions. Let's show a simple example for illustration. In 2022 Kaggle Survey:
- the average annual compensation reported by Data Architects amounted to 93K USD
- the average annual compensation reported by Data Engineers amounted to 49K USD
Based on the above data points, should we conclude that Data Architects earn 90% more than Data Engineers? If we look deeper into the data, we immediately realize that the factor driving the difference is experience: in the said dataset, the median coding experience of Data Architects is 10-20 years, while for Data Engineers it is 3-5 years.
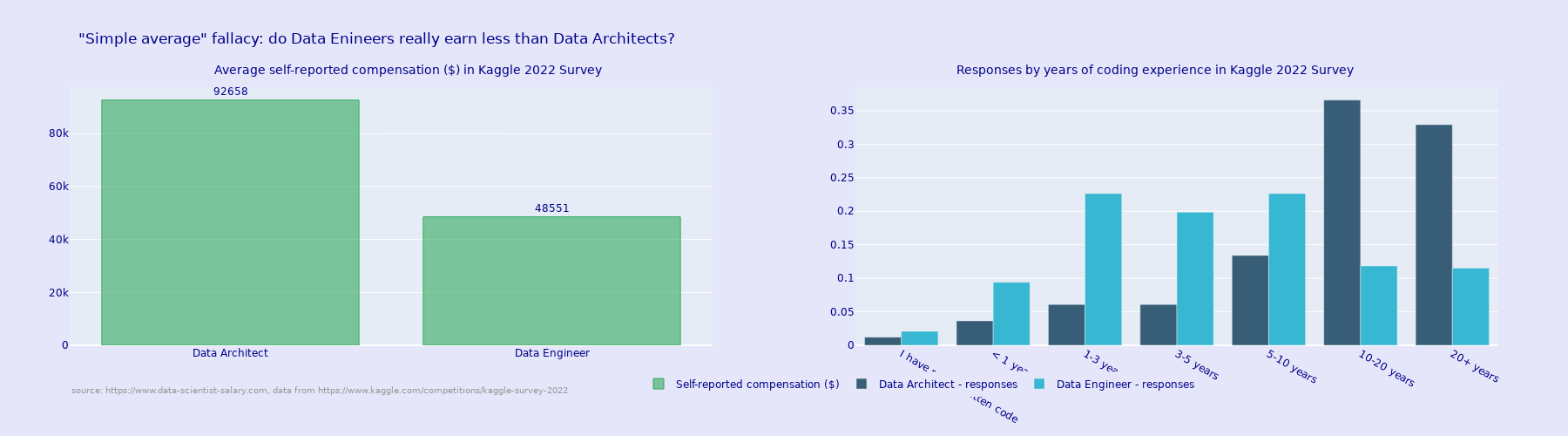
There are infinite possibilities for such a bias to appear in self-reported data, therefore it is impossible to fix manually. The correct approach is to:
- Look at the record-level data and identify key factors impacting the salary of a Data Scientist
- Perform predictions across all combinations of key factors to make sure that each combination appears only once and save it in the "inference table"
- Aggregate the above "inference table" to arrive at the expected Data Scientist salary per the desired dimension
Such is the approach that we took while building our Data Science compensation prediction model.